The Leader in Value Stream Management for Digital Transformation
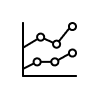
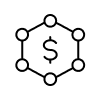
-
Strategy
Connecting ideas to outcomesGet everyone on the same page, finally
Use roadmaps and data to give everyone visibility into the strategy and foster an ongoing, two-way conversation with Business and IT about how to deliver the most customer value—even as situations change.
Turn strategy into a realistic plan for getting work done
Create a clear thread from portfolio-level initiatives to individual work deliverables. When customer needs and plans shift, ValueOps shows what moved, why, and how it impacts other work.
Always access the metrics you need
Persona-based dashboards and views streamline reporting and drive accountability. Everyone has real-time visibility connected from nearly any 3rd party vendor into what’s happening, what’s changed, and what it means to them.
-
Money
Discovering where value is—and isn'tPlan and prioritize investments based on data
Track, manage, and align thousands of work efforts into single value streams. Know what to fund, what it costs, and, most importantly, how it delivers customer value.
Eliminate waste and redundancy
ValueOps empowers you to manage funding at a value stream level. You can optimize investments across different teams without renegotiating budgets for individual work efforts, and resources flow freely to where they're needed most.
Make smarter funding decisions
Monitor and adjust funding based on real-time performance, pivoting proactively to maximize opportunities instead of waiting for the end of a reporting period.
More on ValueOps by Broadcom
-
People
Optimizing teams and resourcesDesigned to work, no matter how you work
ValueOps gives you the best of both worlds: a single platform to manage all demand and work, with the flexibility to let teams keep using the tools and methodologies they’re most productive in. A software should fit into your culture, not the other way around.
Visibility at any scale—and at every level
Easily roll-up data from an infinite number of teams, see how it aligns to specific goals, and understand the dependencies between value streams, teams, and the resources within them. Connect nearly any 3rd party platform into your ValueOps solution for optimized efficiency.
More effective, engaged, and motivated teams
Everyone, from dev teams to the upper management has a consistent view of how their work creates customer value, leading to happier employees, and better decision making at every level.
More on ValueOps by Broadcom
Success StoryHow Chipotle boosted development productivity by 25%
Learn how ValueOps value stream management helped Chipotle drive efficiency throughout the product lifecycle.
ReportUsing VSM to Speed Digital Transformation
Featuring insights from industry analysts and expert VSM practitioners.
-
Work
Improving visibility, accelerating deliveryStop problems before they happen
With ValueOps, you have real-time progress data at your fingertips, at both micro and macro levels. You can be confident you’re on track and make adjustments before small problems cascade into bigger issues.
Data you can trust, insights you can act on
Data and metrics are aggregated from nearly any 3rd party platform in the software development lifecycle into actionable insights — not just numbers — with tailored views, so you can make decisions that power your specific part of the business.
Eliminate Friday night reporting fire drills
Traditionally, reporting progress has been a royal pain. With ValueOps, data is all in one place and easily viewed, so the report you need is just a click away.
Get everyone on the same page, finally
Use roadmaps and data to give everyone visibility into the strategy and foster an ongoing, two-way conversation with Business and IT about how to deliver the most customer value—even as situations change.
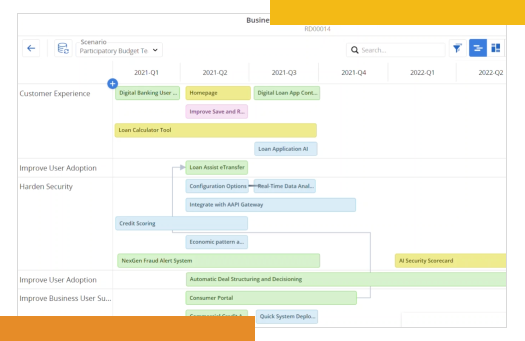
Turn strategy into a realistic plan for getting work done
Create a clear thread from portfolio-level initiatives to individual work deliverables. When customer needs and plans shift, ValueOps shows what moved, why, and how it impacts other work.
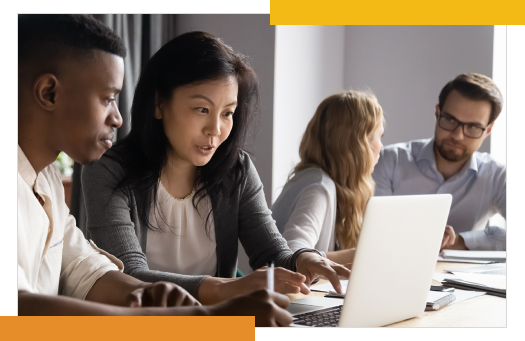
Always access the metrics you need
Persona-based dashboards and views streamline reporting and drive accountability. Everyone has real-time visibility connected from nearly any 3rd party vendor into what’s happening, what’s changed, and what it means to them.
Plan and prioritize investments based on data
Track, manage, and align thousands of work efforts into single value streams. Know what to fund, what it costs, and, most importantly, how it delivers customer value.
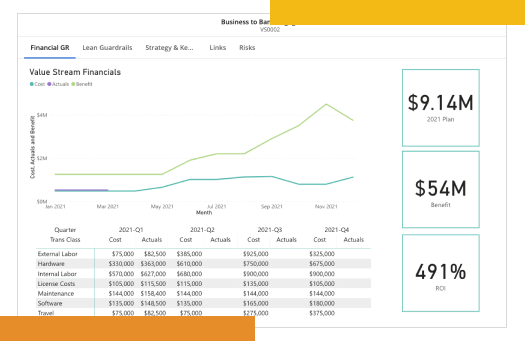
Eliminate waste and redundancy
ValueOps empowers you to manage funding at a value stream level. You can optimize investments across different teams without renegotiating budgets for individual work efforts, and resources flow freely to where they're needed most.
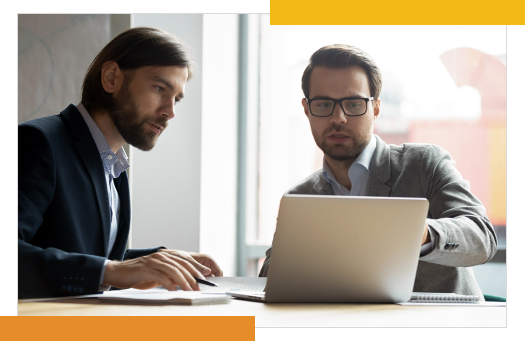
Make smarter funding decisions
Monitor and adjust funding based on real-time performance, pivoting proactively to maximize opportunities instead of waiting for the end of a reporting period.
More on ValueOps by Broadcom
Designed to work, no matter how you work
ValueOps gives you the best of both worlds: a single platform to manage all demand and work, with the flexibility to let teams keep using the tools and methodologies they’re most productive in. A software should fit into your culture, not the other way around.
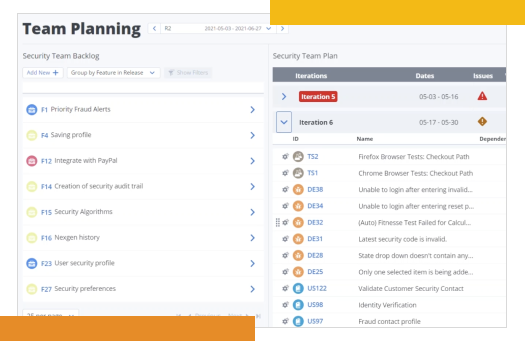
Visibility at any scale—and at every level
Easily roll-up data from an infinite number of teams, see how it aligns to specific goals, and understand the dependencies between value streams, teams, and the resources within them. Connect nearly any 3rd party platform into your ValueOps solution for optimized efficiency.
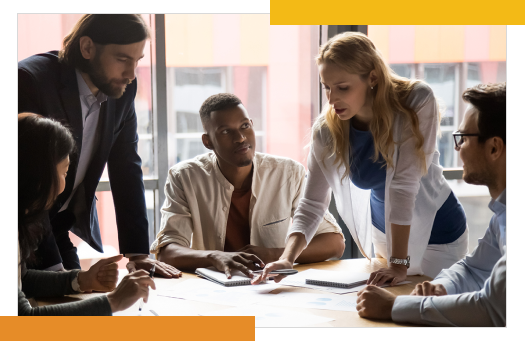
More effective, engaged, and motivated teams
Everyone, from dev teams to the upper management has a consistent view of how their work creates customer value, leading to happier employees, and better decision making at every level.
More on ValueOps by Broadcom
How Chipotle boosted development productivity by 25%
Learn how ValueOps value stream management helped Chipotle drive efficiency throughout the product lifecycle.
Using VSM to Speed Digital Transformation
Featuring insights from industry analysts and expert VSM practitioners.
Stop problems before they happen
With ValueOps, you have real-time progress data at your fingertips, at both micro and macro levels. You can be confident you’re on track and make adjustments before small problems cascade into bigger issues.
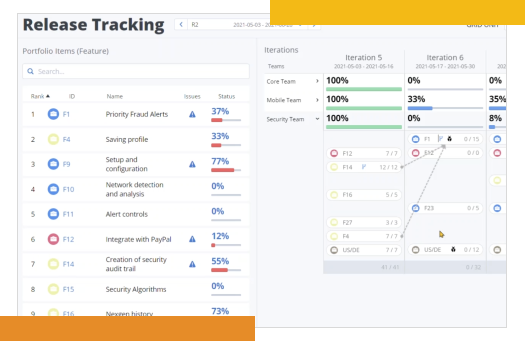
Data you can trust, insights you can act on
Data and metrics are aggregated from nearly any 3rd party platform in the software development lifecycle into actionable insights — not just numbers — with tailored views, so you can make decisions that power your specific part of the business.
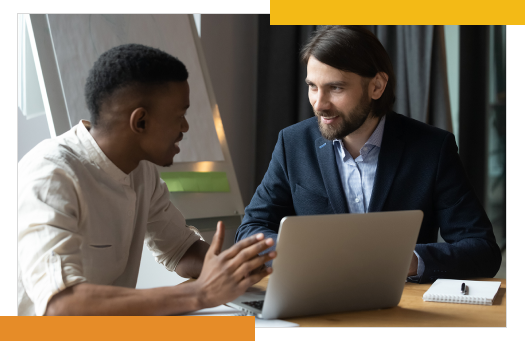
Eliminate Friday night reporting fire drills
Traditionally, reporting progress has been a royal pain. With ValueOps, data is all in one place and easily viewed, so the report you need is just a click away.
“We saved hundreds of millions of dollars in the first two years with ValueOps.”
Program Manager, Portfolio Management Systems
“Executive management is talking the same language as the delivery teams, and ValueOps enables that.”
Product and Process Manager, EPMO
“Our business partners have confidence in their planning decisions and our ability to deliver.”
Associate Vice President, IT Management
“In 2022, we delivered twice as many large initiatives with ValueOps.”
Executive, Office of Value Management and Delivery
“ValueOps is a critical part of how Verizon evolves.”
Senior Manager, Systems Engineering
-
More ValueOps Resources
Quick Start GuideDemystifying Value Stream Management
Learn how to set yourself up for success with VSM- and avoid common pitfalls
StudyThe Total Economic Impact™️ Of ValueOps by Broadcom
Learn how this ValueOps customer in global aerospace manufacturing achieved 471% Return on Investment (ROI) over three years with potential payback of approximately $81.6M in nine months.
-
STUDY
The Total Economic Impact™️ Of ValueOps by Broadcom
Learn how this ValueOps customer in global aerospace manufacturing achieved 471% Return on Investment (ROI) over three years with potential payback of approximately $81.6M in nine months.
More ValueOps Resources
Demystifying Value Stream Management
Learn how to set yourself up for success with VSM- and avoid common pitfalls
The Total Economic Impact™️ Of ValueOps by Broadcom
Learn how this ValueOps customer in global aerospace manufacturing achieved 471% Return on Investment (ROI) over three years with potential payback of approximately $81.6M in nine months.
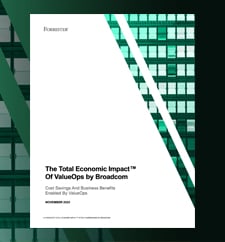
The Total Economic Impact™️ Of ValueOps by Broadcom
Learn how this ValueOps customer in global aerospace manufacturing achieved 471% Return on Investment (ROI) over three years with potential payback of approximately $81.6M in nine months.